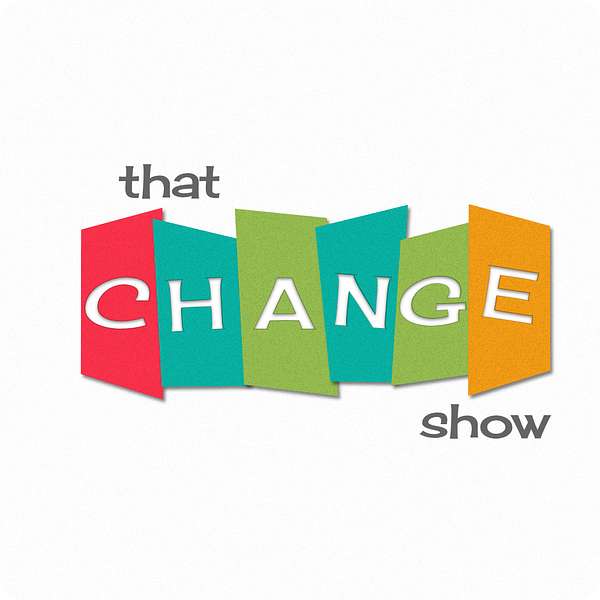
That Change Show
Change management doesn't have to be boring. That Change Show is a weekly-ish, live and unfiltered podcast that dives deep into the messy world of change and transformation. Join Lean Change found and two-time Amazon best selling author, Jason Little as he destroys the status quo while exploring topics like AI's role in change, lean and agile change management techniques, and commentary on the world of change management. If you're an agile coach or change manager craving radical insights, this is your new addiction.
Jason is the author of The Six Big Ideas of Adaptive Organizations, From Skeptic to Strategist: Embracing AI in Change Management, Lean Change Management, and Change Agility. Video episodes available at https://leanchange.tv
That Change Show
AI-Powered Sentiment Analysis and Its Impact on Organizational Change
Have ideas for the show? Liked a topic? Let us know!
Discover how to tap into the emotional pulse of your audience using AI-driven sentiment analysis, a topic we tackle with fervor as Phoebe from Australia joins the conversation. We're not just scratching the surface of customer feedback and employee opinions; we're delving deep into the emotional spectrum that can revolutionize how businesses interpret complex data. Imagine having the ability to discern intricate emotional patterns and leverage this information for impactful change management and strategic decision-making—this episode promises to unlock that potential.
We take you through a journey of understanding, where sentiment analysis is not merely a tool but a gateway to the heart of your organization's most pressing concerns. From the varying attitudes of professionals across different experience levels to the influence of community backgrounds on perceptions, we unpack how sentiment polarity and subjectivity scores can be a game-changer. Listen as we reveal how an analysis refined by AI can guide not only organizational transformations but also shape the readiness for change, providing invaluable insights that are tailor-made to suit the nuanced needs of your team and clientele.
https://leanchange.org/ai
Jason Little is an internationally acclaimed speaker and author of Lean Change Management, Change Agility and Agile Transformation: 4 Steps to Organizational Transformation. That Change Show is a live show where the topics are inspired by Lean Change workshops and lean coffee sessions from around the world. Video versions on Youtube
All right, here we go. Sunday, february 18th. Welcome back to that Chain Show. I'm your host, jason Little. Today I'm going to be chatting about using sentiment analysis and AI. So I did have a podcast scheduled for this morning, but the person I was supposed to be hosting wasn't able to make it today, so I had to call an audible. So what I thought I would do is do something with a little more visuals. So if you're listening to this in your podcast, listener Thingamajiggy, you can go to leanchangetv or you can go to the LeanChange YouTube channel Both links you can find in the show notes and you can check out some of the visuals. I'll do my best to describe them as we're going along. So what I'm going to do for this video is talk about different approaches you can take for collecting insights from customers, from employees.
Speaker 1:Typically, this is going to be in a change management context. So let's just say you're doing some type of readiness assessment, some analysis. You want to get feedback on how the change is going. These are pretty common and what I see with surveys is, a lot of the times we'll overly simplify them to make it easier to analyze the data. So we'll ask things, like you know, from a scale from zero to five, how much do you agree with this statement? We might ask more binary related questions or things like that, because we can get nice fancy charts out of there so we can go. Oh, 73% of the people agree with this change, as rated by most people. Put it anywhere from agree to strongly agree in this.
Speaker 1:So what AI is going to allow us to do is to collect much, much deeper insights for everything from readiness assessments to business readiness to even project information. So if you've got a bunch of status reports and a bunch of other data collected from a variety of sources, you can actually do much, much, much better analysis on that stuff and you might not even have to worry about just doing periodic surveys. You can collect that stuff basically from anywhere. You can dump in a bunch of emails, you can dump in a bunch of tweets, or if you're using any kind of internal social media site or SharePoint or whatever it is that you're using. It's pretty cool because AI doesn't care where the data comes from. So you're going to be more likely to get better insights that are going to help you figure out how to move the change forward. I'm not going to get into a lot about data privacy and ethics, because obviously that's going to be a big problem, especially if you're talking about collecting insights from email. So I'm going to do probably another show on ethics later on down the road, but let's get into this one. So what I'm going to do for this one, I'm going to use the state of AI and change management survey for some of the examples. I'll do that a little bit later in the chat and again, if you're listening to this in your podcast listener thingy, I'll describe the prompts that I'm putting in and how it's responding.
Speaker 1:But first let's talk a little bit about the term sentiment analysis. Now, from what I see mostly on LinkedIn and forums and conversations and stuff, sentiment analysis typically gets represented as positive, neutral, negative. I wanna do a sentiment analysis on this text and we oversimplify our ask of AI to basically say you know who agrees, who disagrees, who's somewhere in the middle. There's a whole bunch of different techniques that you can use for sentiment analysis. I'm gonna do a little quick definition of the nine main ones and a little bit of the differences between the two, because the interesting thing with AI is, if you just asked it, for example, can you do a sentiment analysis on blah and you paste in a bunch of text.
Speaker 1:It is going to default to a certain model and it's gonna give you insights. So basically, yes, it is going to do the analysis, but it's gonna do what you ask of it. Sometimes it might not be able to analyze the data. It might ask you some questions to be able to prepare your data and stuff like that. But if you wanna get a bit of deeper analysis, you can use one of these nine techniques. Okay, so, instead of describing these myself, I've invited a friend of mine from Australia, phoebe, to describe this stuff for me. So how's it going today, phoebe?
Speaker 2:I'm doing great today, although I'm a little disappointed. Volkanovsky lost the title last night.
Speaker 1:Yeah, I know. Yeah, it was a good fight. Maybe he came back a little bit too soon, but he's still one of the greatest.
Speaker 2:Thanks, I appreciate that.
Speaker 1:Yeah, no problem, I think he's gonna get a rematch anyway, probably in Spain, which would actually be pretty cool. But anyway, the reason why I asked you here today was to explain in a really simple way the different types of sentiment analysis that people can do on text. So could you take like all the major ones and just maybe explain it in, I don't know, a couple of sentences for each?
Speaker 2:Certainly that sounds like a cracking idea. I'll give it a go. Artificial intelligence is revolutionizing how we understand our customers and employees. In the past, we would rely on rating scales, agree to disagree sliders and simplified questions, because it was easier to see a chart about the percentage of people who strongly disagree versus those that don't. In a world, teaming with data, unlocking the nuanced emotions behind texts is key to truly grasping what people want and need. From tweets to reviews, survey responses to casual chats, every word shared online or in the workplace carries a wealth of information. But how do we decode these vast textual landscapes to discern true feelings and opinions? Enter AI and its groundbreaking tools making sense of sentiments at an unprecedented scale.
Speaker 2:In this explainer video, we'll unravel the mysteries of text preprocessing keyword density analysis, bag of words, tfi-df, sentiment lexicons, machine learning models, transfer learning with pre-trained models, veda and TextBlob. Each technique, a unique lens magnifying the whispers and shouts of human emotions hidden within texts. Don't worry if you don't know what those terms are. Keep watching and we'll explain its simple terms. When we're done, you'll be able to give chat GPT your data and ask it to use one of these nine techniques text preprocessing. This is like cleaning up and organizing a messy room before you start decorating. It involves getting the text ready for analysis by removing unnecessary words, correcting spelling and breaking the text into smaller parts.
Speaker 2:Keyword density analysis imagine you're looking for clues in a detective story by noting how often certain words appear. This technique counts how frequently specific words related to feelings or opinions show up in the text, giving a basic idea of the overall sentiment Bag of words bow. Think of it as making a grocery list from recipes. You list all the unique ingredients words you need without worrying about the order they appear in the recipe text. This helps in understanding which words are common and might be important. Tf-idf term frequency inverse document frequency. This is like deciding the importance of an ingredient based on how often it's used in a recipe and how unique it is across all recipes. It helps figure out which words are not only common but also uniquely significant to certain texts.
Speaker 2:Sentiment lexicons imagine you have a dictionary where every word is tagged with a mood label happy, sad, angry. This method uses such a dictionary to scan the text and determine the overall mood based on the words used. Machine learning models this is akin to teaching a computer to recognize patterns in a pile of mixed up socks by showing it examples of which socks match. Over time, the computer learns to sort new socks into pairs positive, negative, neutral sentiments on its own Transfer learning with pre-trained models. Imagine if you could download a chef's cooking skills into your brain. Transfer learning lets a computer model do something similar, where it learns from a vast amount of data someone else collected, making it smarter at understanding text sentiment without starting from scratch.
Speaker 2:Vader. Think of Vader as a specialized tool for reading short messages like tweets or reviews and quickly deciding if the message sounds positive, negative or neutral, taking into account slang, emoticons and other internet specific expressions. Text blob picture a helpful assistant that reads through text and gives it a happiness score. Text blob is easy to use and good for getting a quick sense of whether the text is generally positive, negative or neutral. Remember AI is only effective based on how you prompt it, prepare it for what you want to accomplish with the analysis, who your audience is, the questions you've asked and how you want the data broken down. And remember, chat GPT remembers the context so you can follow up with questions like are you sure? Can you reanalyze that? Can you analyze that data using the bag of words approach. There is no longer a need to take the easy way out with numerical rating scales. The possibilities are endless, and your creativity will be the most important factor for deeply understanding your customers and employees.
Speaker 1:Oh, that was great. Oh, that was so awesome. Thanks very much.
Speaker 2:You are most welcome.
Speaker 1:I did notice one thing, though your lip sync is a little bit off, Are you? You know? Is there some latency going on with your internet over there?
Speaker 2:I know, but I think you were just too lazy to ask me to create a better lip synced video.
Speaker 1:Yeah, fair enough. Okay, so for now, this is a custom GPT. If you go to chatopenaicom, you will need to have a plus, a GPT plus account to access this. We're going to be integrating this into the website anyway and all of our research that we've been doing over the last 15 years. So this is the state of AI and change management and our ongoing surveys and random questions we've asked over time so you can ask this a specific question about how many people responded to the AI survey. I think we're at about 41 or two, I think, so far, and it should pick it out. It might take it a little bit to analyze it, but okay, so we've got 42.
Speaker 1:Now, if we're starting off with sentiment analysis, let's just start with the easy question. So I'm going to ask it can you just do a sentiment analysis on the AI survey and just tell me the general sentiment? So let's see what this comes up with. By default, chat GPT is going to default to the Vader mode, which is you could call it a fancy keyword search, so it's going to rip out all of the oh no. So here's an interesting thing If it messes up, it's going to try again, which is pretty cool. So I made some assumptions about the existence of comments column for the sentiment analysis, but it didn't align, so let me try this again. So now it's actually read the questions. So this is if you remember chatting about the importance of cleaning up your room before you get started, this is a CSV output of the data. The column headers have the questions in it and I just fed it the new data this morning. So it does need to take a look at that stuff first so I can understand it. So what I should have done is I should have asked it this. So now, if you're asking it, you basically you have to ask it if it understands the data. So now it has the questions. Now I can basically do more deeper sentiment sentiment analysis on these types of questions. But let's just scroll up to the first section up here.
Speaker 1:Now, what I did up here can you do a sentiment analysis on the AI survey only and tell me the sentiment? This is called zero shot prompting. So this is basically you're giving chat GPT a prompt or, sorry, an instruction to do something that it's never done before. So it's like if you had a brand new employee who was super duper smart and you said go do this thing. It's never done it before. It's going to go try it and it's going to base it on its experience, which is why it said it appears I made an assumption about, so it assumed there would be a comment column. Now if I was doing something called few shot prompting, now I would do a question like this, I would say tell me what questions were asked in the survey, or I'm gonna upload a file or just text of my survey. Can you tell me whether or not you understand it and what other questions you have? That's few shot prompting. So basically you're gonna train it with a little bit of information before you get started. So if I go back to the zero shot prompt here and highlight this, so the survey indicates general positive sentiment towards AI, with a polarity score of approximately 0.18. So it says that the tone is slightly positive. The subjectivity scores around 0.48, meaning that there is a moderate level of subjectivity in the responses. So it does also recognize that there's personal opinions and feelings in the responses and there's also a significant amount of objective discussion. So not too terribly useful. But now it knows the questions.
Speaker 1:If I asked it again, consider the question about how people are confused by AI only, and can you analyze those responses and summarize them into key themes or categories? So it should only take those questions. The really good thing about doing this kind of analysis is you can ask the AI I'm not sure that's correct, because you're gonna know, generally speaking, what type of data, questions and insights you wanna get out of it. So you can say I'm not entirely sure that's correct, can you try again? So now it's breaking things down into themes or categories. So if you're listening to this, the main themes were ethics and integration with people and what is AI's role in change management, risk in the future of AI, application and policies, tools and access. So it's plunking out the major themes from what are people confused about?
Speaker 1:Now, the interesting thing again with the sentiment analysis is this is just from experience. When you ask people questions in a survey, sometimes their brain just kind of goes down a dirt road and they put in data that's not really related to the question. So the good thing with this is I can ask it now to create that same theme, but across all of the different types of questions. But what I wanna focus on here is breaking things down, because some of the things that we're talking about with sentiment analysis is at scale. So what I did with this question was I asked people about their years of experience and basically what community they came from, because I wanted to see if there was a difference in perception between agile coaches, change managers, hr people or other. I also asked about technical aptitudes so not technical at all from still developing in writing code. So now I can slice and dice these types of things by asking questions like is there a difference in sentiment between experienced people with 10 or more years of experience compared to people with less than 10 years of experience? And when I asked this question on the survey, it wasn't a range, it was basically just use a slider from zero to 20. And then assuming if it's 20, then obviously it would be 20 or more years. So now it's using something called train of thought response and it's telling me what it's gonna do. So it says the years of experience in change management among the respondents range from two to 20 years. So it makes it straightforward to categorize these answers. So now it is giving me the answers. So experienced respondents 10 or more.
Speaker 1:The sentiment has a polarity of 0.12, indicating a slightly positive tone in their skepticism. The subjectivity score is around 0.46. And when it is less experienced change agents, the sentiment is more positive, with a polarity of 0.2 and more subjective, with the subjectivity score of 0.6. Now suppose you don't know what those mean and I'm not gonna pretend to be a statistical expert. So I'm gonna ask it and I'm gonna say what does the polarity score mean? So now it's telling me it says the polarity score is a numerical value ranging from negative one to positive one and it's the overall sentiment. So negative values is basically the text is expressing a negative sentiment. The closer the score is to negative one, the more negative it is. Zero is neutral, positive is zero to one. So that's pretty interesting. So you can see 0.12 is a lot closer to zero than 0.2. So that's how it's telling me that less experienced people have a more positive view of what AI is.
Speaker 1:And now I can also ask it the subjectivity score, which I am assuming is gonna be the same. So now it's gonna respond with zero indicates the text is very objective, with no opinion or emotion, and one is very subjective. So it's basically a zero to one scale. So you can see that the answer had had above. The subjectivity score was 0.46 for experienced people and 0.6 for people with less experience. Now, because it stays in context, if I wanted to see if there was a difference between communities. Now so I can say can you do the same analysis again? But instead of analyzing it by experience, can you break it down by community? So now it's telling me again train of thought.
Speaker 1:Response the respondents belonging to sorry, the respondents belong to three distinct communities Agile, change management and other. Now I did ask for HR and stuff like that, but just from memory I don't think anybody obviously answered that they identify or that, sorry, they're more closely aligned with the HR community. Otherwise it would have had that. So now it's spitting out the polarity and the subjectivity per community. Now my assumption going into this was Agile people would be more accepting of AI because, generally speaking, they are more likely to adopt early technologies. Now there might be some bias because we're kind of in the corporate metuism stage or phase of Agile, which is, people are getting brought into Agile nowadays through an enterprise lens or through scaling frameworks and certifications and things like that. So there might be a little bit of bias in. It would be interesting if I ask people when did you get involved in the Agile community Because the people who were involved very early on tend to be more on the innovative curve and more early adopters. Change management, in general terms, is generally more conservative and the polarity that it came up with here. So the positive sentiment of Agile was 0.22 and change management 0.11 and other community 0.05, so slightly positive and other could be obviously just about anything. Now, the subjectivity Agile community was 0.51 and change management was 0.45. So the summary that it comes up with you know obviously you can see it on the screen, but if you're listening in your podcast listener thingy, the Agile community has the most positive sentiment towards AI in change management.
Speaker 1:Now I wanna do the exact same thing, but now this is the last one I'm gonna do, because I think you get the point of what you can do here, but I want to break it down by technical level. So, while it's thinking about this, the reason why I wanted to slice and dice these this way because now you can sort of figure out what do you wanna do with those insights. So if you were doing an analysis like this in your organization for a transformation or a change or whatever it is, and you could, I did this the hard way. Once I don't know 12 years ago or something like that where we had data from probably 600 people. We sliced it by department and we basically wanted to see what was the thought, you know, what was the readiness level, if you wanna call it that of the change from the perspective of different departments.
Speaker 1:And we found out jeez, I really gotta dig into the memory archives for this one but there were some groups that were much, much, much more accepting of the agile transformation that we were working on than other groups. Now, it wasn't a case of, oh, we need to go to those other groups who rated a low score and we need to overcome the resistance and focus on them. What I use that for was well, let's forget about those guys. Let's go with the people that are motivated and wanna do this stuff, let's latch onto them, let's figure out how to help them as much as they need, and then now they're gonna be the movers, they're gonna be the people who help the other people who are not ready for this yet. Come along for the ride, because those movers or innovators, or early adopters, however you wanna call it those are the people who are most likely to want to be coached, and we certainly did have teams in that organization that said give us everything you know. Just, we're ready for coaching, we're ready to try stuff out, we're ready to take on the extra work, because everybody's got project schedules to meet and stuff like that, and we didn't bother with the teams that you know from the survey didn't really want any help. Eventually they came around.
Speaker 1:So now let's take a look at the exact same analysis, and my assumption here was I have to think about what my assumption was. It's on the screen and I've already seen it, so I'm gonna try to not let that sway my decision. I thought people who were more technical would be more excited about it, and the reason I say that is there's so many sit with a developer and talk with a developer about all the manual things you do and they can probably automate or use AI for stuff and make your lives dramatically easier, and whenever I've done that with people, they get blown away. So I do this in my startup, where I put this in the ultimate guide to prompting book. The founder of the startup that I worked for, that I'm still working for, wanted to organize a conference, and I basically did this with ChatGPT. In about 10 minutes we went from a big flip chart of topics people wanted to talk about from an autism meeting that she had done to a full schedule with suggested profiles for speakers to get. And she was totally blown away because this sticky had been sitting in our office, this gigantic sticky wall, for probably a year and a bit, and every time we would go by and we would look at it. We're like one of these days we're gonna look at that and we're gonna try to make sense of it and organize a conference. And now, once she saw how easy it was to do this, it just like the possibilities in her mind just completely exploded.
Speaker 1:So I thought the same thing would happen here. The technical people would be more likely to know how much AI can help them in change. Again, because these technical people they're probably agile coaches or technical coaches, former developers, stuff like that and I thought the people who were less technical would be like, oh, whatever, it's just a fancy search engine. So let's see what the sentiment is Currently developing. Things was the question for people who were highly technical. They had a 0.18, positivity sentiment and people who were not technical at all had a negative 0.16. So, woo-hoo hypothesis validated, I guess, people who did basic things. So basically everybody had a positive sentiment, except for people who were not technical and they had very low subjectivity scores. So this data all right. So that's it for this week's edition of that change show.
Speaker 2:Join us next week because we're gonna be talking about a really interesting topic Are enterprises finally ready for agile development? So there's been some talk about what Bear is doing and I'm surprised it hasn't completely exploded on LinkedIn. As agile folks like to latch on to these things have more deep knowledge and experience with change compared to agile people. Ken Rucker is gonna be joining me for that one and we're gonna be chatting about is this the first domino that?
Speaker 1:needs to fall for enterprises to really generalize agile transformation.
Speaker 2:So again, check out nchangetv. And those are just the power of our episodes and the video versions. Agile folks tend to like to shine things faster.
Speaker 1:Hit, like and subscribe if you're watching this on YouTube and we will tend to take more of a wait and see kind of approach and maybe a more conservative approach. So both of those things are gonna be important when it comes to getting thrown into AI projects. All right, so let's get into the wrap up. So, now that we've done a few things with the sentiment analysis, if one of those other nine techniques sounded interesting to get you more insights, you can specifically ask it. You can say can you reanalyze that using the TFIDF approach? It's gonna spit out a list of its term. Frequency, basically, is what that one is, so it's gonna pluck out the number of times those terms were found in whatever responses you're analyzing. Now that might not be too terribly useful. If you were using this for product development, for example, and you put feature names in there, that might be more useful, because now you can start to see what features are people talking about, which would be pretty interesting. So if there were specific parameters about the change that had those kind of keywords, you could use that analysis on it as well, which would be pretty cool.
Speaker 1:But I think the lesson here is we don't have to rely on single shot surveys, which is every quarter. We do an employee employee feedback survey. You can do ongoing ones, because now you can also ask AI to say tell me what's changed over the last year? Can you break down the responses month by month and spit that out in a fancy chart so you can feed it random questions basically anytime, so you don't have to get the questions right, and I see this a lot with change, especially when you're talking about enterprise change. You get these surveys that take you 20 minutes to fill out and by the end the employees are just like I don't care, I don't care, I just want this thing over so I can get back to my cat videos on Instagram. So you don't have to rely on those gigantic surveys at the beginning. You can take data and you can add it at any point in time, which is really awesome. So I hope you enjoyed this video. Drop a comment below if you've got questions.
Speaker 1:Next week I am going to be bringing Ken record back. We're gonna be chatting about our enterprises now ready for agile transformation. This is based on the stuff that we're seeing about bear what they're doing, so the reader's digest version is they're getting rid of their management layer, flattening the organization and using a different approach for how they're doing transformation, which in a company like that is pretty impressive, and the hypothesis for the chat in the next episode is is this the first domino that needs to fall for enterprises to really get what agile transformation is all about? So remember to hit like and subscribe on our YouTube channel or in your podcast listener thingy, and I will see you next week. Thanks for watching.